In the 2017 Economic Survey, the official annual statistical Bible of India, the finance ministry indirectly admits that its figures related to GDP (economic) growth may be wonky. In Chapter 3, which focuses on demonetisation, it states: “It is clear that recorded GDP growth in the second half of FY 2017 (demonetisation period) will understate the overall impact” of the ban on high-denomination notes. The reason: the government has no idea about what happens in the unorganised or informal sector, which contributes to half or more of GDP.
The Survey states that chunks of the “informal or cash-based” economy are “not captured in the national income accounts”. To the extent that a few sections are measured, it is done on an approximate basis. The Survey admits it is based on “formal sector indicators” and it is assumed, for no apparent and logical reason or justification, that the informal segments of India’s economy behave the same way as the organised counterparts. In specific terms, the Survey contends that “informal manufacturing is proxied by the Index of Industrial Production, which includes mostly large establishments”.
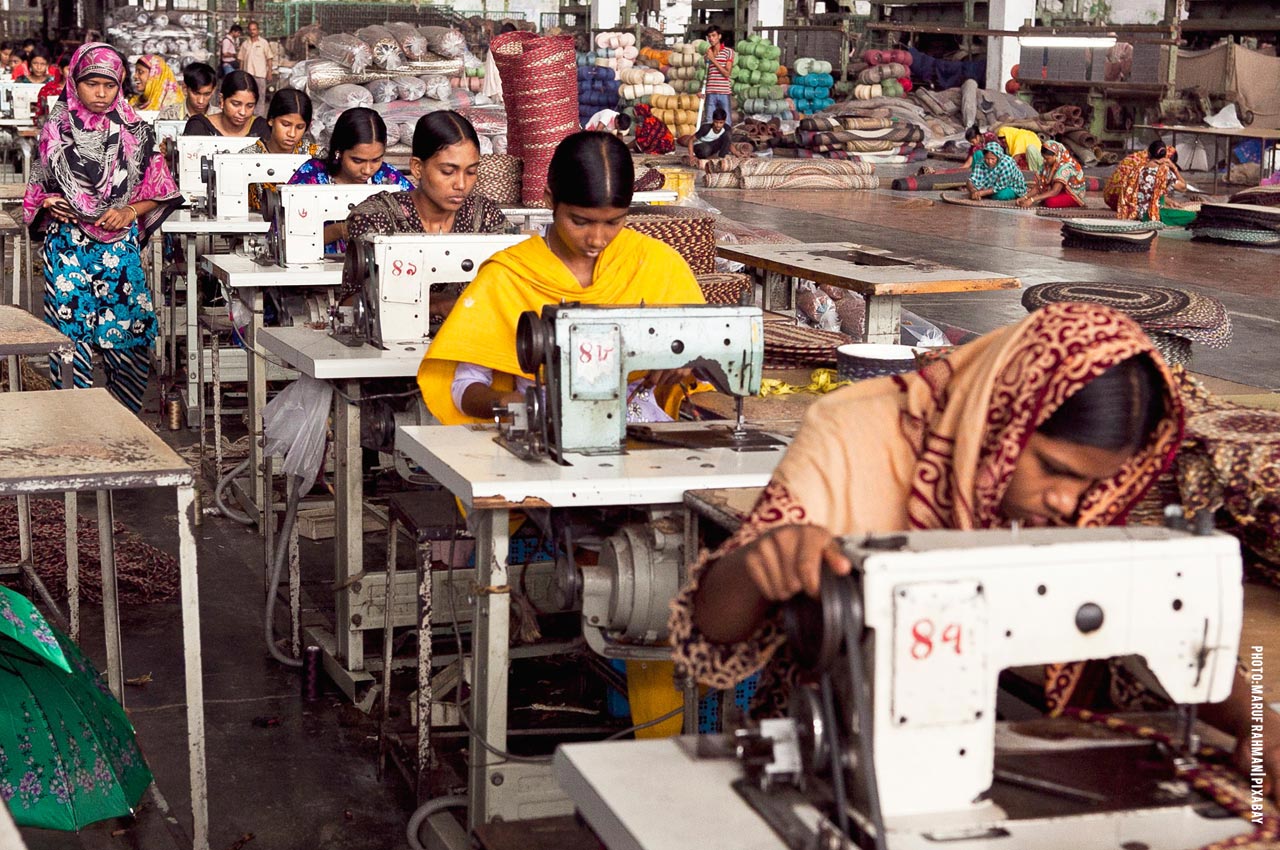
What this implies is that the government thinks that the occurrences in the unaccounted for markets mirror those in the organised ones. More important, if there are economic earthquakes in the unorganised segments, as was the case during demonetisation, the hasty and messy implementation of the Goods and Services Tax (GST), the pre-Covid slowdown, and finally the pandemic, the government can only partially measure the impacts. This is especially true if such events have a more negative effect on the informal sector, compared to the formal one, which is true for the above-mentioned cases.
Companies pay taxes on their profits, and not revenues. As comparable data shows, overall corporate revenues continue to dip, which indicates economic inactivity
For those with implicit faith in official data, who take it at face value and use it consistently for several purposes, the above admission is a virtual slap in the face. If the GDP figures, which are the basic and fundamental numbers for any economy, cannot be trusted, what can be? The 2017 Survey puts huge question marks on every statistic that is doled out by the various ministries. It is clearly a case of half-baked figures that are released as economic truths. We have heard that statistics can lie, and in this case, they evidently do.
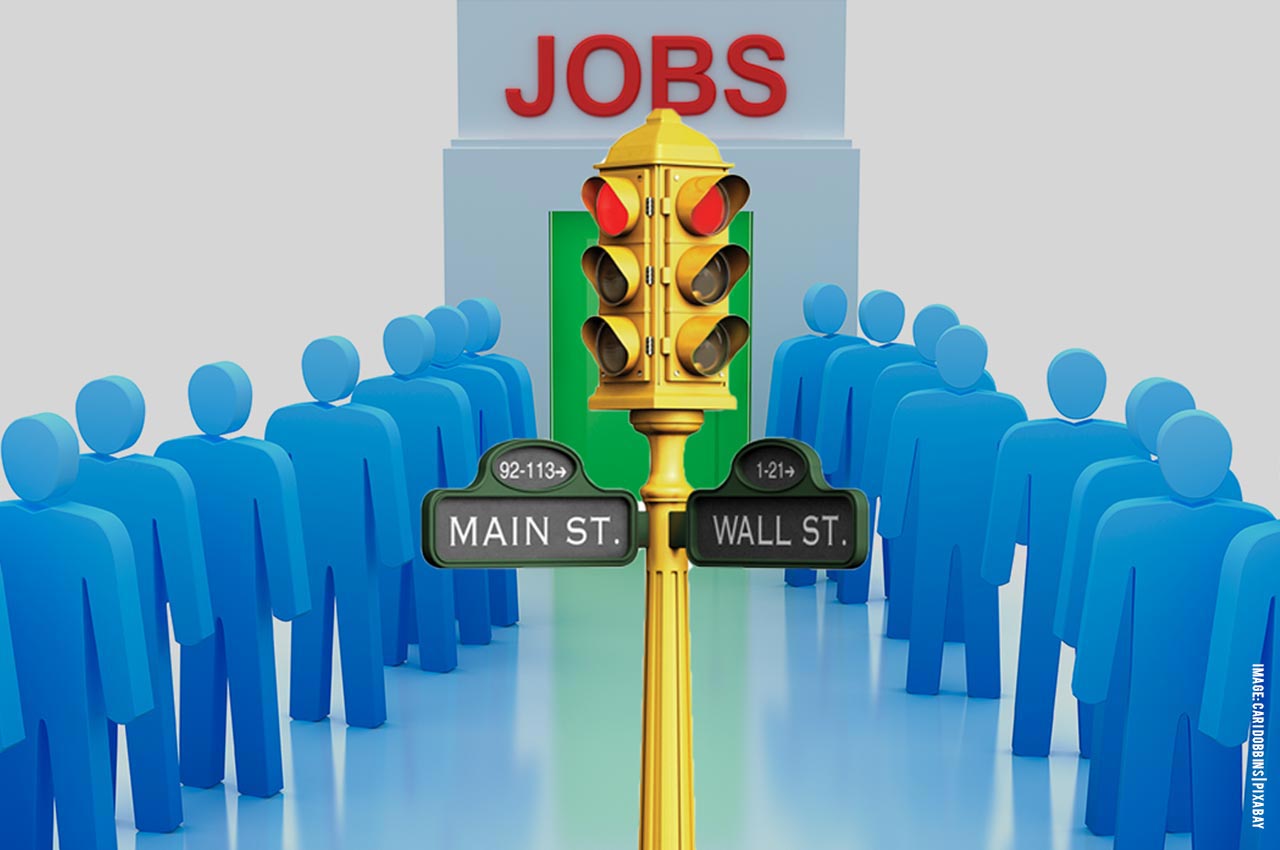
Let’s take another case of how data is massaged. After the second wave of the Covid-19 pandemic killed tens of thousands of Indians, the finance ministry put out figures to prove that the economy was still healthy. The numbers show that net direct tax revenues, which is the government’s income from individual taxes that you and I pay, and corporate taxes, between April 1 and June 15 this year were an “astounding” 100% more than the figure for the same period in 2020. GST collections remain robust; they topped over ₹1 trillion a month for the past eight months.
On the face of it, the figures are impressive. Tax collections in these three areas indicate that individual and corporate incomes bounced back, as did consumption. Net direct taxes confirm the first contention, and GST the second. But there are a few catches. The first is that companies pay taxes on their profits, and not revenues. As comparable data shows, overall corporate revenues continue to dip, which indicates economic inactivity. The profits zoomed, thanks to cuts in expenses like marketing and manpower. Hence, companies paid more taxes.
Several factors can explain the higher-than-expected GST. One relates to inflation. The RBI’s annual report (2020-21) states that the price rise during the year is the highest since 2013-14, and is largely fuelled by the food and beverages segment. As we know, the prices of vegetables and fruits are up, and so is the case with vegetable oils. In some cities, the price of petrol is higher than that of a bottle of beer. Since taxes like GST are charged as percentages of the prices of goods, like 10 percent of ₹100, their collections go up as prices move northwards.
In both cases, the companies and consumers suffer—the first due to low revenues and the second due to higher prices. But the manner in which the government presents the tax data seems to hint that everything is rosy with the economy, the corporate sector, and the citizenry. Unlike the problem of measurement, which affects GDP, the major issue with tax collections lies in inadequate, subjective, and unexplained cause-and-effect linkages. Higher corporate taxes and GST may not necessarily indicate better production and boom in purchases. They are certainly not the best indicators of the overall health of the economy.
Unemployment figures are the best way to understand the intricacies of data, and what they do and don’t capture. First, it needs to be mentioned that when the unemployment numbers went awry, and rose in the pre-Covid slowdown phase, the government put a stop to their release. We had to base our assessments on what was released by think tanks, global agencies, and private institutions. In fact, even now, we do not have a fair idea of how many millions lost their jobs, either permanently or temporarily, due to the pandemic.
What is relevant is the manner in which unemployment is calculated. It shows how distorted the figures can get, and how we may forever be fooled by what they indicate and imply. Most of us feel that it is a simple formula—the percentage of people who don’t have jobs out of the total population in the working age group. This is untrue. Unemployment rate is actually the percentage of people without work out of the total population in the working age group “that seeks jobs”. If you and I don’t want a job, and we don’t have one, we are not unemployed.
Now, this becomes an interesting and insightful exercise. The formula excludes sizable sections such as house-workers (male or female) and students (who may be in the working age group). Excluded from the calculation are people who stay at home for one reason or another, and even some of the casual and temporary workers who don’t have regular jobs. If you are not in the active job market, and if you don’t have a job, you are not unemployed, and are not considered as one. The choice, desire, and intention on the part of the jobseeker is crucial.
In technical jargon, the denominator in the formula is dubbed as the labour force participation, or the percentage of the working age group that wishes to be in the job market. Obviously, there are compelling reasons to complicate the calculation. A house-worker cannot be considered unemployed since he does not want to be part of the employed. To label them as people without jobs is unfair to them. Similarly, if a youngster wishes to pursue higher studies, she cannot be included in the unemployed category.
Since taxes like GST are charged as percentages of the prices of goods, like 10 percent of ₹100, their collections go up as prices move northwards
However, the scenario becomes frightening if, over the years, the percentage of the working age group population, which shuns and avoids jobs, inexplicably increases. This means that more people opt out of the job market, either deliberately because they cannot get one, or under pressure from their spouses and families. The socio-economic dynamics in such situations don’t augur well for any economy. It reduces and depresses the number of productive people—house-workers are contributors in several ways, but remain unpaid—in the country.
Economists call this phenomenon vanishing labour. In India, unfortunately, such cases have zoomed over the past several years. This is visible across age groups, in both urban and rural areas, across gender, and among most of the socio-economic sections. More importantly, it is most prominent among women, who prefer to stay at home, and out of active jobs for various social and economic reasons. In some states, the figures are particularly damning, and reflect a lot of things that are wrong with past governments’ governance strategies and policies.
Between 2005 and 2018, the number of women in the 15 to 59 age group who sought jobs, plunged by a steep 20 percent due to several reasons. During the same period, the percentage in the middle-aged 30 to 59 bracket who shunned active work, spiked from 46 percent to 65 percent. It means that only a third of them were either employed or interested in jobs. The scenario was more dismal in 2018, as three-fourths of rural women and four-fifths of their urban counterparts, remained out of the labour market. This is bad news for any economy and country, and its policymakers.
Compare India’s figures with other nations, developed and developing, and one gets an idea of the malaise. The percentage of women’s participation in the labour market is high in the western world—Switzerland (80 percent), Japan (73 percent), and the US (68 percent). A blog by researcher Deepa Krishnan states that the numbers are growing in nations like Bangladesh, where the percentage shot up from 24 percent in 1991 to 36 percent in 2017. It is unbelievable that in this aspect India finds itself among the bottom 10 nations, with only nine in the Middle East and Africa below it.
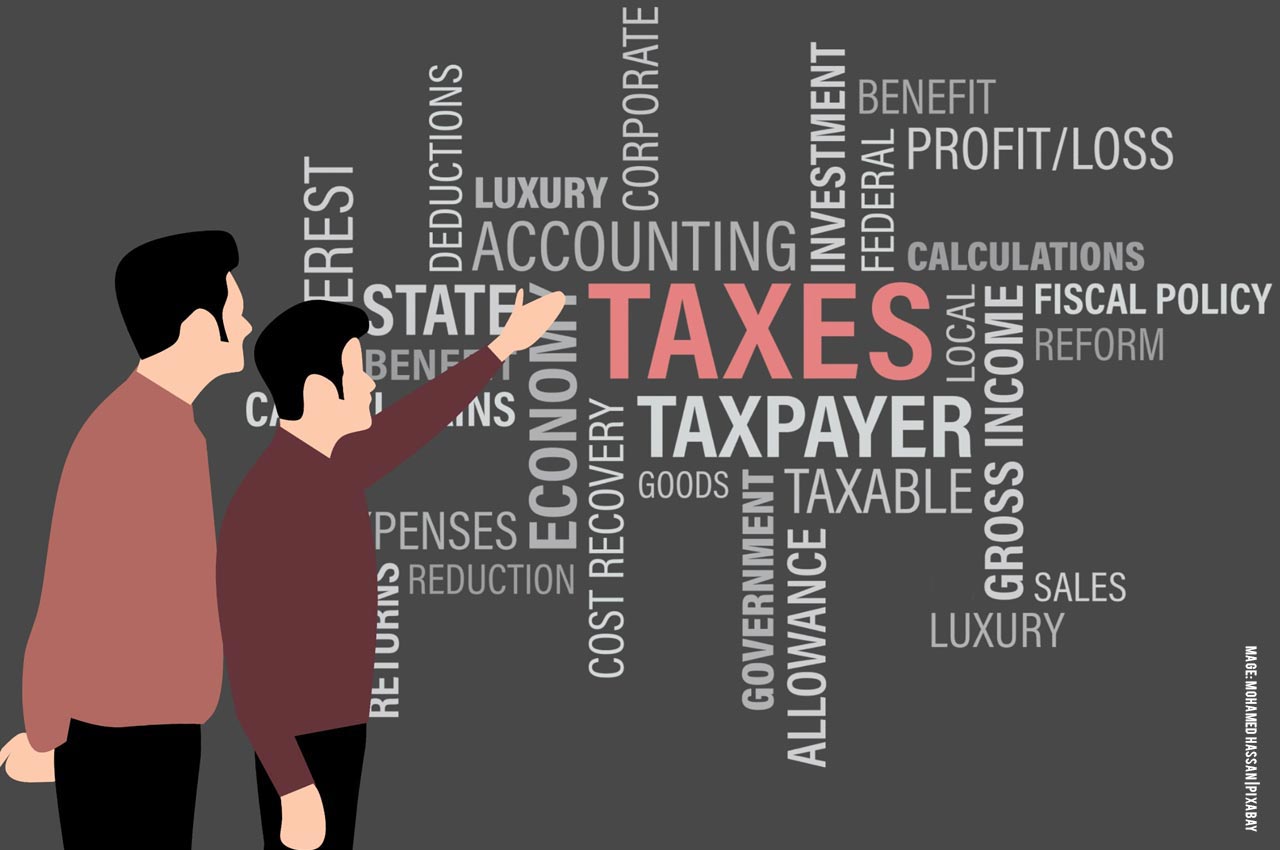
Between 2005 and 2018, the number of women in the 15 to 59 age group who sought jobs plunged by a steep 20 percent due to several reasons. Compare India’s figures with other nations
Let us turn our attention to what’s happening in some of India’s states. According to the figures for 2017-18, the participation of rural women in the labour market was abysmally low—under four percent in Bihar and only 14 to 15 percent in Uttar Pradesh (UP), West Bengal, Punjab and Haryana. Shockingly, this is the state of affairs in UP and West Bengal, which witnessed especially high GDP growth rates in the recent past. Both Punjab and Haryana are considered more progressive and prosperous states in socio-economic terms.
In an appraisal of the above data, the negative connotations stand out. But since statistics are generally double-edged swords, cutting both ways, the same figures lead to positive conclusions. Since lower percentages of women in the working population desire jobs, the unemployment rates in the five states are low. The figures for jobless females are 1.5 percent in UP, 1.7 percent in West Bengal, and 2.3 percent in Bihar. This isn’t because of the states’ policies, but due to the calculations. The choice of the denominator impacts the numerator and depresses the percentages.
So, the next time you look at socio-economic data, digest it with plenty of salt. The measurement may be wrong or partially true. The links between the figures may not reflect cause-and-effect. And most of the numbers can be seen either as a half-filled glass or a half-empty one. These are the major issues with official data. Unless we, as consumers of figures, are aware of them, we will never get the complete picture. Now pick up the Economic Survey with caution and circumspection.
By Alam Srinivas